Navigating The Hype Of AI In Clinical Research
By Sarah Valentine, partnerships manager, life sciences, Digital Medicine Society (DiMe)
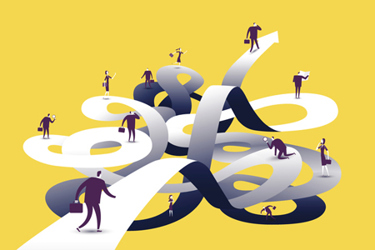
Chances are, you’ve seen something on ChatGPT in the past six months. If your LinkedIn looks anything like mine, you probably can’t get through a minute of scrolling without seeing posts hyping up how generative AI will revolutionize healthcare. And do you know what? Some of the hype is true.
Imagine leveraging AI to write your trial protocol, giving your clinical teams the much-needed time and space to focus on more strategic work. Or imagine using AI to automatically clean continuous streams of data coming from disparate data sources, freeing up capacity on the data engineering side of the house to build out broader integrations with real-world data and bridge the gap between clinical development and commercial use cases. AI could even become the investigational product itself, as a digital coach or an element within a combination product.
Opportunities to leverage AI in clinical research are limitless. What you might not realize is, these examples aren’t the future of clinical research. They’re the current state.
AI is here today and it’s here to stay. And I think that’s a good thing. When you consider the rising cost of healthcare in America, a shortage amounting to tens of thousands of physicians, and economic headwinds stirring fear across the field, we can’t afford to not use these tools. We shouldn’t be asking ourselves questions of why, but rather, why not.
But that begs a new question: Why haven’t we seen the impact, adoption, and value of AI more broadly across clinical research?
Sure, there’s been concern over the state of regulations. But with that concern has come quite a bit of movement in this space. Earlier this year, the White House secured commitments from several leading tech companies to lay the foundation for the safe, secure, and transparent development of AI, and the FDA released draft guidance to support companies developing AI and ML enabled products by establishing guardrails for predetermined change control plans. There have been several developments in the EU, and while there’s opportunity for further alignment, it feels like we’ve struck the match, in a sense, and new regulations will continue to guide the way for innovative AI-enabled products.
We’ve also seen advances across industry through collaborative efforts. In the past year, the Coalition for Health AI (CHAI) released an initial draft blueprint for trustworthy AI implementation guidance and assurance for healthcare, and it has a number of other frameworks that dive into elements of credible, fair, and transparent health AI systems. The Digital Medicine Society (DiMe) released toolkits to help developers of AI navigate the regulatory landscape within the U.S. Partnerships at the intersection of healthcare and technology, like the recent partnership announced by AWS and Eversana, continue to blossom across the field to pave the way forward for pharma, CROs, product developers, and other stakeholders within our field.
But all of these guidances, frameworks, and toolkits are specifically designed to support innovators at the cutting edge, where there’s a lack of precedence or where it’s unclear how regulations might apply. Not all AI is created equally. Other forms of AI exist in our industry today. Why haven’t we reaped value from these tools? Why haven’t they been adopted?
Are we even ready to get the most we can out of AI? Frankly, I think the answer is no.
I’m recalling a stat that colleagues at the Tufts Center for the Study of Drug Development shared earlier this year – that the average time it takes a single organization to move from pilot phase to implementation phase is six years and, beyond that, the average time it takes our field to achieve full-scale implementation (business as usual) is 20 years.
Knowing this, I think a better question we can ask ourselves today is, how can we prepare for the broad adoption and scale of AI — for when AI becomes business as usual? I think the answer comes in three parts.
First, we have to keep blazing the trail forward. Those of us at the cutting edge must continue to ask the hard questions and put in the research to determine how we can best leverage these technologies to augment the important work that each and every one of us conducts in the day-to-day, in a way that’s ethical, effective, equitable, and safe.
Second, we have to continue blazing the trail together. Regulators can’t write guidances for gaps they aren’t aware of. Companies can’t take advantage of new benefits if they don’t know those benefits even exist. Engaging in precompetitive collaborations and discussions that span multiple disciplines will be critically important to ensure comprehensive best practices are established for these current and future tools.
And third, we need to educate ourselves. It goes beyond just reading an article on LinkedIn. Read up on CHAI frameworks to hear how leaders on the bleeding edge think about these topics. Take courses in the Digital Medicine Academy to better understand the value of AI to drug development, ethical considerations for AI, and how AI tools might be regulated. Use tools that exist today to build your skillset so you can see past the hype and identify trustworthy products and partners.
Maybe it’s not as easy as scrolling through LinkedIn for a few minutes each morning. But to the patients we exist to serve, preparing ourselves for what’s possible and how we can enable faster access to lifesaving therapies is well worth the time.
About The Author:
Sarah Valentine leads partnerships across life sciences at the Digital Medicine Society (DiMe). In her role, she thinks critically about the challenges we face in drug development and commercialization to drive strategy, prioritization, and concept development across areas of unmet need where there's opportunity at the intersection of healthcare and technology to deliver unprecedented value to patients and other stakeholders across our industry. She convenes teams of interdisciplinary thought leaders and subject matter experts to tackle some of the biggest challenges we face as a field in order to advance the ethical, effective, equitable, and safe use of digital medicine to redefine healthcare and improve lives.
Prior to her role at DiMe, Sarah was a digital implementation lead at Eli Lilly & Company, where she led efforts at the intersection of clinical development and digital health to leverage innovative digital technologies including digital measures, combination products, and other DDTs in clinical research.