AI And Life Sciences: How Much Disclosure Is Enough?
By David McCombs, Vincent Shier, Eugene Goryunov, Dina Blikshteyn, and Brooke Cohen
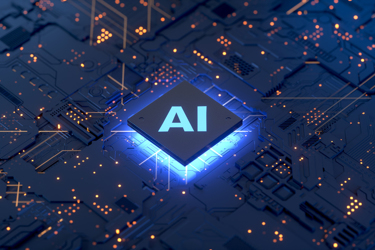
Under the quid pro quo of the patent system, inventors are given a 20-year exclusionary right over their inventions in return for disclosing to the public sufficient details about their invention. Through this exchange, inventors are rewarded for investing time and resources into advancing the science. At the same time, science continues to advance as a result of the enabling disclosure.
However, is this bargain worth it? The answer is nuanced. Companies must first decide whether getting a valid patent will require too much disclosure such that it would negatively affect commercializing the invention. Indeed, disclosure usually leads to new competition and a competitor could easily design around the invention and produce a similar, competing product.
Extending this analysis to patenting artificial intelligence (AI) inventions is especially challenging. AI inventions are often “black boxes” that receive data and generate a result. During training, these “black boxes” manipulate the weights given to the training data to increase the accuracy of the program. Moreover, these “black boxes” are often not front facing, and instead are stored securely within the companies’ servers. Accordingly, unless a company publicly discloses the type of a neural network or the algorithm that its AI uses, the AI inventions, weights given to training data, and internal optimizations are difficult to copy or reverse engineer. Thus, companies may favor maintaining their AI inventions a trade secret rather than disclosing it in patents.
As tempting as a trade secret route is, however, it may be impractical in AI for drug discovery, therapeutic target identification, or other aspects relevant to pharmaceuticals or medical device technologies. Companies that choose the patent route must decide how much disclosure is enough to satisfy the patent disclosure requirements.
In our previous articles, we discussed how life sciences inventions incorporating AI face hurdles getting patented for lack or inventorship or for being directed towards patent ineligible subject matter. In this article, we focus on what it takes to meet the enablement and written description requirements of 35 U.S.C. § 112(a) when patenting AI inventions in life sciences.
Juggling the requirements of § 112(a)
At first, section 112(a) may look like a kraken with too many tentacles. However, a savvy practitioner can effectively manage its requirements while at the same time creating a specification that is as clear and concise as possible.
Two of the most daunting requirements of § 112(a) are similar, yet distinct: enablement and written description.
Enablement
Enablement requires that the specification “enable any person skilled in the art [POSITA] . . . to make and use” the patented invention.1 The patentee must provide enough information in the specification such that a POSITA can practice the claimed invention without “undue experimentation.”2
Whether or not the claimed invention is enabled depends on several different factors, particularly whether the patent relates to an unpredictable art, such as chemistry or the life sciences.3,4
Satisfying the enablement requirement can be particularly challenging for AI-driven inventions in the life sciences. It can be difficult to understand exactly what disclosure is required for a POSITA to recreate the “black box” of the AI program covered by the patent. Right now, Congress and the courts do not offer much guidance. AI is a new technology, so the case law is still evolving.
However, the first step of enablement is to understand and define the level of expertise of the POSITA. For innovations in this technology, the POSITA would need to understand both the AI program itself as well as the biological or biochemical principles underpinning the program. Thus, the POSITA for AI-driven life sciences innovations would require experience in distinct areas: life sciences and computer science – which is relatively uncommon.
Written Description
Although related to enablement, written description requires that the specification “contain a written description… in such full, clear, concise, and exact terms” of the patented invention.5 The specification must “reasonably convey[] to those skilled in the art that the inventor had possession of the claimed subject matter as of the filing date."6 Thus, written description requires that the invention be described such that a POSITA would recognize that the inventors actually conceived of the invention covered by the patent application by the filing date. Enablement on the other hand requires that the specification provide enough detail so that a POSITA can make and use the invention.7
Strategies for disclosing exactly what is required-nothing more, nothing less
Striking a balance between keeping certain information private, on the one hand, while satisfying the many requirements of § 112(a), on the other, is a daunting task. However, there are techniques that ensure that the proper balance is struck.
At the outset, practitioners should consider who the POSITA would be. To determine this, practitioners can ask: What is the field of the invention? Does the person need computer and life sciences backgrounds? If so, how many years of experience should such person have in each discipline? Is a graduate degree required and why? By understanding more about who the POSITA is, practitioners can tailor the specification to that person and avoid significant § 112(a) issues down the road.
Second, a practitioner can include discrete blocks that explain the general structure of the AI program in the specification. If possible, including a pseudo-code of an algorithm the AI program uses may also be helpful. The practitioner can describe the blocks, algorithms, and/or pseudo-code and how they fit together. In many cases, it may be advantageous to give examples of structures that perform the operation and how the data flows from structure to structure. When a neural network is used, the practitioner can include the layers of the neural network model, the inputs, the outputs, and how the data is manipulated within the neural network model itself.
Practitioners should be wary of making the blocks too generic. For example, a questionnaire for collecting information from a clinical trial participant is often interpreted using natural language processing models, such as BERT and GPT-3. In these cases, the specification should include more description than the generic BERT or GPT-3 structure. A good specification might include a discussion of how the data from the questionnaire flows through the structure. A great one might explain how the data flows, is acted upon, and is changed by different components from the structure. If the structures are too vague, the specification is likely to encounter enablement issues during examination and in validity challenges in later litigation.
Third, it may be helpful to include information about the training data in the specification. For example, for an AI program that determines toxicity of a potential compound, training data describing the structure of known toxic chemicals and the particular biological targets (e.g., cellular receptor) that the toxic chemicals interact with might be helpful. Because AI programs are trained or fine-tuned on training data to make future predictions, the training data may need to be disclosed so that the enablement and written description requirements are satisfied.
Fourth, when the invention is an AI program that predicts pharmaceutical compounds, practitioners should aim to identify and claim a broad genus that covers the most likely drug compound and any similar compounds that could potentially have a pharmacological effect. As many exemplar compounds as possible may be included in the specification to have the proper support for the genus. Thus, practitioners may need to reach out to the inventors and ask for more information or encourage them to identify a common chemical structure or characteristics that can be used to support a genus claim. This may involve using the AI program to output additional compounds.
Fifth, another strategy to consider is describing an AI program as one of many tools. For example, AI may be listed in the specification as one of many tools for performing a certain analysis. Even though this may work for some inventions, typically, more information may be necessary for a POSITA to practice the invention. Therefore, when framing the AI program as a tool, practitioners should consider including parameters, weights, and structures of the AI program along with the training data that is being used.
Sixth, the practitioner should ensure that multiple aspects of the invention are covered by a variety of claims. Including different types of claims and varying claim scope can increase the likelihood that at least some of the claims withstand § 112(a) challenges. Practitioners should be mindful that it is better for the specification to support broad, useful claims. AI is a new technology and the field is not crowded yet. Thus, the patent examiners often issue broad claims for AI inventions. When the specification has the right mix of high-level overviews and relevant details about the invention, practitioners can seek patents that have broader claims.
With the right balance, patents for AI and life sciences driven innovations are valuable
Striking the right balance between sufficient disclosure while keeping the specifics of the invention a secret can be difficult. However, there are strategies for ensuring that both the AI program itself and the pharmaceutical or diagnostic method resulting from AI receive valuable and robust patent protection.
The authors are attorneys at Haynes Boone, LLP.
This article reflects only the present personal considerations, opinions, and/or views of the authors, which should not be attributed to any of the authors’ current or prior law firm(s) or former or present clients.
- 35 U.S.C. § 112(a).
- In re Wands, 858 F.2d 731, 736-37 (Fed. Cir. 1988).
- Id at 737.
- There are eight factors that courts analyze when deciding whether the invention is enabled: “(1) the quantity of experimentation necessary, (2) the amount of direction or guidance presented, (3) the presence or absence of working examples, (4) the nature of the invention, (5) the state of the prior art, (6) the relative skill of those in the art, (7) the predictability or unpredictability of the art, and (8) the breadth of the claims.” Id.
- 35 U.S.C. § 112(a).
- Ariad Pharms., Inc. v. Eli Lilly & Co., 598 F.3d 1336, 1351 (Fed. Cir. 2010).
- Along with enablement and written description, § 112(a) also requires the best mode of the invention be disclosed in the specification. 35 U.S.C. § 112(a). Although best mode cannot be the basis of holding a claim invalid and only rarely arises in certain post-grant proceedings, the best mode still needs to be disclosed to comply with the law. 35 U.S.C. § 282(b)(3)(A). This requirement may concern companies that want the best embodiment of their invention private; however, a good patent will protect the best embodiment rather than allow others to copy it.