AI In A Time Of Uncertainty: Key Strategies To Enable Flexibility
By Gaugarin Oliver, founder and CEO, CapeStart
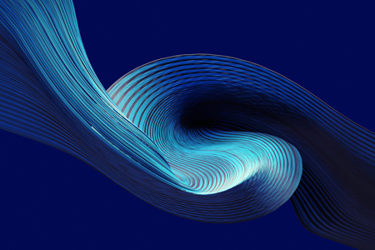
The onset of the new U.S. presidential administration brings with it sweeping changes to U.S. policy related to artificial intelligence (AI). As a first step, the Trump administration revoked the Biden administration’s Safe, Secure, and Trustworthy Development and Use of Artificial Intelligence, an executive order providing guidance for the creation of AI solutions. Though the new administration has hinted at AI policy that provides more freedom for innovators, this sea change is creating disruption and uncertainty in the near term.
In addition to uncertainty in policy, the speed of innovation for AI has accelerated dramatically, causing chaos in the AI market. China’s DeepSeek created a comparable AI model to ChatGPT at a fraction of the cost — just $5.6 million compared to the hundreds of millions spent on US-built models — and just days later topped this breakthrough with an even cheaper Alibaba AI model.
Despite the uncertainty, interest from life sciences leaders in AI is high, with 2024 data showing that 91% of life science leaders believe in AI’s value, and three out of four are either using, testing, or actively deploying an AI-enabled application in their operations.
Belief In AI’s Value Among Life Science Leaders
The combination of demand for AI solutions, unknown risks, and the unique pressures on life science leaders — whose decisions can impact patient outcomes — all make AI development a challenging environment to navigate. When considering these complexities, life science leaders need AI strategies that instill flexibility in their AI development and solutions, allowing them to stay nimble.
Key Strategies For Flexibility In AI Development
There are four key strategies for enabling flexibility in AI development that life science leaders should consider when initiating AI solutions. These are:
- Implementing a modular design and flexible architecture for solutions.
- Establishing an environment of continuous learning and upskilling for AI teammates.
- Maintaining high-security awareness and adaptation to ensure secure systems and best practices for vigilance in security.
- Insisting on high standards around privacy protections, staying compliant with global privacy policies, and training staff regularly as a matter of practice.
With thoughtful application, these recommendations can enable a flexible AI development path for life science leaders.
Modular Design And Flexible Architecture
With AI models and innovations evolving at a breakneck pace, rigid, monolithic systems are virtually obsolete. In this environment, modular design and flexible architecture are important platform and product elements for AI systems because modularity and flexible architecture allow for easy updates and modifications without requiring complete system overhauls. The ability to swap in different large language models (LLMs) — for example, Google Gemini, DeepSeek, and open-source models — while maintaining a stable core structure is essential for systems to maintain flexibility. Minimizing disruption in life sciences is critical as neither time nor quality can be squandered in the drug development process.
Modularization in AI systems supports several aspects of drug development. For example, a life sciences company may use AI-driven molecular modeling to accelerate drug discovery. Depending on which part of the process is under consideration for AI support, a different LLM might make sense. For statistical numeric extraction, an AI team might use Claude, but for synthesizing clinical publications ChatGPT 4.0 might be more appropriate. The complexities of drug development require this level of flexibility to keep up with model innovation. This approach allows life sciences companies to rapidly adopt the latest LLMs and enhance drug candidate predictions without disrupting their entire system.
This dynamic approach accelerates the process while also enhancing collaboration between different LLMs trained for different tasks, optimizing the available innovation to bring the greatest value to its AI solution and AI use cases. Beyond model selection, independent modules also can include data ingestion, decision-making logic, and user interfaces, among other capabilities.
Flexible architecture for AI development includes Retrieval-Augmented Generation (RAG) and Agentic AI. RAG allows AI to pull real-time, relevant external data while ensuring data gathered remains up to date with the latest information. Agentic AI helps automate specific granular workflows and combine them as required to keep decision-making adaptable to new insights and shifts in policy. Testing and benchmarking across models are key to maintaining flexible architecture. As a best practice, golden data sets (or ground truth data) provide important benchmarks for testing new AI models before implementation. Tools like LangFlow and LangFuse track performance and enable the efficient comparison of different AI frameworks. This type of AI assessment and validation is important in life sciences not just for the general quality of the AI output, but also for regulatory compliance around AI usage.
Continuous Learning And Upskilling
To effectively deliver AI solutions, AI teams must stay updated on the rapidly evolving AI landscape, where new models and innovations emerge monthly, if not more frequently. This is especially true in life sciences where the benefits AI can bring — mainly time and quality — have the potential to accelerate and improve multiple steps from molecule to medication, with the overarching goal of improving patient outcomes. Ultimately, awareness and understanding of AI innovations and regulatory guidance enable greater creativity in addressing situations that require flexible, innovative solutions. As such, life science leaders should invest in ongoing learning and ensure their teams are equipped to evaluate, test, and integrate new AI technologies regularly.
Teams should prioritize AI innovation as a core component of company strategy. Recent data shows that more than 80% of life sciences companies have included objectives around AI in their goals. Many are currently focused on delivering AI solutions that help them improve efficiency and shorten aspects of the drug development process. As part of this prioritization, look to assess AI advancements based on their business impact for the life science organization, regulatory requirement support, and overall feasibility before implementing.
Organizational Goals For AI And Performance Improvement
Having a dedicated R&D team to explore advancements and prioritize AI implementations is essential to maintaining flexibility in AI development. These resources should be cross-functional, continuously learning and sharing new AI insights across the organization. From the cross-functional team, leaders should establish an internal research unit tasked with tracking new AI developments, best practices, and FDA guidance. Set internal goals around testing emerging AI models and innovations in the space before any life science implementations to minimize risk.
Teams also should create an internal culture around AI training, growth, and knowledge sharing — keeping it oriented to life sciences to further your industry expertise. Invite clients or external stakeholders to join in where appropriate. For example, if conducting a comparison of a new model against an existing one, teams should debrief important external stakeholders in addition to sharing the analysis with the internal team. Showcase the company’s focus on AI learning as a point of differentiation and celebrate the team’s success as they grow in this expertise.
Building a knowledge-sharing organizational structure to support this dynamic learning is important as well. Create internal AI knowledge repositories where teams share best practices and lessons learned from their AI projects. Establish regular AI knowledge-sharing sessions with cross-functional resources to constantly be sharing new ideas across the organization.
Security Awareness And Adaptation
As AI systems become more integrated into business operations, security risks, compliance challenges, and regulatory scrutiny increase. To mitigate these issues and ensure compliance with heightened regulatory demands, life science leaders need to integrate security frameworks, guardrails, and governance into their AI deployments. Proactive security governance will help ensure security settings and compliance rules adjust automatically as regulations evolve. Consider including security-by-design principles as part of the AI model-building process, as well as incorporating agent-based guardrails in the AI development process. These efforts will allow the flexibility needed to keep AI security up to date with regulatory changes.
Confidence in AI comes from traceability and explainability around AI decision making. To meet regulatory expectations and security standards, effective AI solutions need to have traceability, transparency, and human oversight. Providing clear explanations of how decisions are made by the AI system is vital to ensuring regulatory approval for the system’s use in the process.
Human oversight is a critical component of AI security as well. In areas of sensitive decision-making, like life sciences and healthcare, Human-in-the-Loop (HITL) configuration, assessment, and validation are essential to managing high-risk actions. HITL practices also enable greater flexibility in pivoting security measures in response to evolving threats, ensuring AI solutions remain robust and compliant.
To maintain security, AI infrastructure should follow zero trust principles, which means that every AI request or action is verified before execution. At the hardware level, System on Chip (SoC) security frameworks also can provide AI-powered security.
By monitoring and proactively adapting to evolving AI regulations, AI systems can enable nimble responses to such regulations as the EU AI Act and the U.S. federal guidelines. This systematic approach to AI security has real-world impact. AI solutions remain secure and compliant with regulations, avoiding potential fines or data breaches. With this strategy, security teams are aligned with AI developers, which ensures that AI advancements do not introduce vulnerabilities to the organization.
Privacy Protection
One can hardly discuss security for AI development without acknowledging privacy policies as equally important. Looming and continuous cybersecurity threats target AI models and infrastructure, requiring robust privacy and security measures.
As AI systems become deeply integrated into healthcare, life sciences, and other regulated industries, security and privacy risks grow exponentially. Life science organizations must ensure that AI models are secure, privacy-compliant, and capable of adapting to evolving regulations and threats.
Data privacy regulations like GDPR, HIPAA, CCPA, and the EU AI Act, require strict handling of patient and personal data and provide guidance for organizations to ensure their compliance. Implementing strategies that protect personal data through privacy-by-design principles (like anonymization and differential privacy) will help ensure compliance and provide flexibility in responding to policy changes without disruption.
Creating Future-Proof AI Systems
Given the near-constant technological advancements in AI, potential AI policy updates, and regulatory changes, flexible AI systems are necessary for life science organizations to keep pace with change. Embracing AI development strategies around architecture, learning, security, and privacy as part of a larger approach to ensuring flexibility will help life science leaders better navigate the uncertainty facing AI development in the life sciences industry.
About The Author:
Gaugarin (“G”) Oliver is the founder and CEO of CapeStart, Inc., a professional services firm supporting the healthcare and life sciences industry, and maker of the award-winning MadeAi™ platform that helps pharma organizations compete and win in the AI economy.