Correct But Misleading: AI Hallucinations In Complex Decision-Making
By Vincenzo Gioia and Remco Jan Geukes Foppen
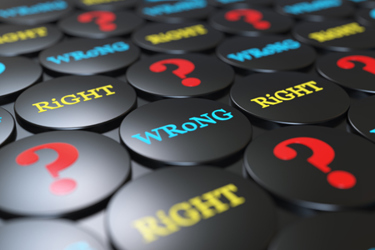
In the increasingly complex world of artificial intelligence (AI), the concept of "correct outcomes" takes on a profound and sometimes ambiguous meaning. Although efforts are made to create AI that produces accurate outcomes, these systems present a complex reliability problem identified as “hallucination.”
The phenomenon of hallucinations in AI systems raises fundamental questions about their capabilities and the risks associated with their use. Hallucinations in AI systems raise serious concerns because they impact the reliability and safety of said systems, especially when they are used for critical tasks such as medical diagnosis, financial operations, or cobotics [collaborative robotics].
We previously discussed the phenomenon of hallucinations in the pharmaceutical sector, with regard to prediction of clinical progress and asset evaluation, and strategic decision-making. Those articles provide a description of the concept and characteristics of this phenomenon. Here we describe an additional point of view on hallucinations inherent to the nature of the responses, and aimed at understanding whether a result or outcome, however correct, coherent and acceptable, could be the result of a hallucination.
How Do Hallucinations Manifest In AI systems?
Hallucinations in AI systems can manifest in different ways, each of which presents unique challenges in terms of verifying the correctness of the response and taking actions to manage the manifestation of the problem. Hallucinations usually manifest as misinterpretations of input data, generation of incorrect information, inconsistent responses, unpredictable behavior, or unwanted sensitivity to certain inputs.
Provability And Probability: Explainability Explained
Explainability deals with the implementation of transparency and traceability in probabilistic black‐box machine learning methods in a biomedical domain. There are promising developments toward white-box methods, like the model Claude 3.5 Sonnet developed by Anthropic.
BenevolentAI is an example within the biomedical domain. They adopt explainable large language models (LLMs) for target prediction and have a potentially first-in-class AI-designed drug candidate in clinical trials. Similarly, investors with or without domain expertise can benefit from explainability with regards to asset ranking.
There is some ambiguity in how to define explainability and what impact it could have. What business impact would explainability have, if one uses a common or logical definition of explainability? One would expect a proof statement. AI explainability (xAI), on the other hand, is based on probabilities, and is a powerful tool to point toward the reasoning, or data points, that underlies an outcome.
Explainability In The Validation Processes Of Outcomes
The management of insidious scenarios created by hallucinations in AI systems can be, in our opinion, simplified by adopting explainability.
Explainability is an important tool when it comes to evaluating responses generated by AI models. It can help determine whether the AI's decision-making process is robust, understandable, and free of any unwanted deviations.
Explainability allows you to interpret "why" and "how" a certain response was generated, offering transparency in the functioning of the model by providing a clear vision of the steps that the model followed to arrive at an answer. Explainability can identify patterns or behaviors of the model that are inconsistent with training data or logical expectations.
Could xAI be an anti-hallucination measure? On its own, explainability would not recognize, or correct, a hallucination. The good news is that a ground truth statement in the data set could be an initial indicator of a hallucination. It is advisable to adopt multiple strategies to evaluate the pathway from prompt to output.
Risks Associated With AI Hallucinations
Hallucinations in AI systems pose a significant risk when such systems are employed in important decision-making processes. This problem is even more complex to manage when hallucinations impact innovation processes or the creation of new products. AI systems used in the pharma sector for the development of new drugs are a prime example. In this context, no matter how sophisticated the control processes of the responses generated by the AI system may be, it is difficult to understand whether a new molecule responds to the characteristics declared by the AI without a verification process. However, due to the elevated volume of solutions that AI can produce, it is not efficient to think of activating a process for every molecule developed.
Explainability In Pharma
Explainability in the context of the pharmaceutical market makes it possible to simplify the explanation and interpretation of the results obtained from AI systems by verifying aspects related to patient safety, transparency in development and testing processes, effectiveness, regulatory compliance, and trust of stakeholders.
Patient safety is protected through the prediction of drug effects with particular attention to the identification of potential adverse effects. The ability to explain how a model arrives at certain conclusions is crucial to ensuring that patients receive safe and effective care. Transparency in the development and testing processes of a drug is mandated by regulatory agencies including the FDA and the EMA, which require explainable AI models to demonstrate that the decisions made are based on solid scientific data and reliable methodologies.
Finally, explainability allows us to improve acceptance and the level of trust among all stakeholders such as doctors, patients, and researchers by showing that the models are reliable, free of bias, and based on understandable logic.
Hallucinations And Correct Outcomes
We are inclined to think that hallucinations only occur when the model generates incorrect or invented responses that do not match the training data or reality. In practice, if an AI system provides a response that satisfies the requirements of adherence to training data, validity, and accuracy, we can consider this response free from the effects of hallucinations.
In essence, consider the risk that even a correct outcome may be affected by hallucination, because it is theoretically possible that a correct result is obtained through an incorrect path. This phenomenon is often due to randomness or coincidence and can be the result of an elaboration process based on an incorrect understanding or on uncorrelated data. An example of random correctness is when someone answers a question based on a false hypothesis but coincidentally arrives at the correct answer. A correct answer also can be the consequence of lucky generalizations which, although not adequate for all aspects of reality, still produce the right answer in a particular case.
In our opinion, the most difficult scenarios to manage are linked to compensatory errors. Compensatory errors occur when two or more distinct errors which, propagating through calculations or processes, can interact in ways that cancel (compensate) each other leading to a correct response. Compensatory errors are not the result of intentional logic, but rather coincidence, and can occur in complex systems where many variables interact and errors can propagate in unpredictable ways.
Thinking that compensatory errors are a false problem is not a reliable strategy because, although they can lead to correct results, they are not predictable and do not guarantee long-term accuracy. Furthermore, compensatory errors raise questions about the nature of knowledge because they generate correct results without correct understanding.
In summary, although incorrect reasoning does not guarantee either a correct or an incorrect outcome, it can occasionally lead to a right answer. However, relying on faulty reasoning is risky and unreliable as a way to solve problems or to make strategic decisions.
Verify Outcomes
The possible presence of hallucinations even in correct outcomes underlines the importance of implementing rigorous and reliable verification procedures to evaluate the consistency and reliability of the answers generated by AI systems. Even if an answer might be technically correct, the presence of hallucinations could compromise its practical usefulness and safety. Therefore, it is essential to develop strategies that allow hallucinations to be identified and corrected, even when they occur in responses considered "correct." These strategies cannot ignore the use of an explainability method.
About The Authors:
Remco Jan Geukes Foppen is an international business executive in AI and life sciences, with proven expertise in the life science and pharma industry. He led commercial and business initiatives in image analysis, data management, bioinformatics, clinical trial data analysis using machine learning, and federated learning for a variety of companies. Remco Foppen has a Ph.D. in biology and holds a master’s degree in chemistry, both at the University of Amsterdam.
Vincenzo Gioia is business and technology executive, with a 20-year focus on quality and precision for the commercialization of innovative tools. He specializes in artificial intelligence applied to image analysis, business intelligence, and excellence. His focus on the human element of technology applications has led to high rates of solution implementation. He holds a master’s degree from University of Salerno in political sciences and marketing.