Demystifying AI Governance: A Strategic Approach For Life Sciences
By Michael Lucas, CISSP, Kristen Bednarczyk, and Luke Pillarella
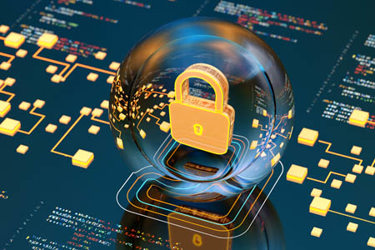
Artificial intelligence (AI) is propelling the life sciences industry into a future filled with potential, where the rapid interpretation of complex biological data, the acceleration of drug discovery timelines, and the personalization of treatment strategies are becoming the new norm. This technological revolution is not just about innovation; it’s about reimagining the way we approach health and wellness on a granular level, with AI as the driving force.
As we explore this new territory, we must tread carefully. The rapid advancement of AI technology brings several risks that must be managed. These risks are not novel but are the same challenges that have been scrutinized and addressed over the past two decades. Therefore, as AI uses solidify roles for life sciences organizations, it is imperative to continue to refine risk management strategies, drawing on the lessons learned from years of managing and monitoring similar risks in other domains.
AI Use Cases In Life Sciences
Early cancer detection. AI models have shown promise in early cancer detection. For example, AI systems have been effective in detecting tiny tumor lesions in breast cancer screenings that could otherwise be missed by radiologists. In addition, researchers at the Massachusetts Institute of Technology developed a model that uses low-dose computed tomography images to predict the risk of patients developing lung cancer.
Drug discovery and development. Once people train AI systems on biological data, the technology can identify potential drug candidates at a much faster rate than was possible before, and it can predict efficacy and side effects with impressive accuracy.
Key Risks For AI Governance
The use of AI technologies in the life sciences industry, while exciting, also creates significant risks given the sensitive information involved and the sometimes life-and-death realities. Effective governance establishes guardrails to enable people to use and develop AI responsibly, minimizing harm and mitigating risk. The following are key risks AI use can present to companies leveraging it:
Data privacy and security. AI models can permanently retain all information that is fed into them. For life sciences companies, which maintain sensitive personal information, it is critical that patients are given the opportunity to affirmatively consent to the use of their personal information and data prior to its use in AI systems.
Bias and fairness. Bias has emerged as one of the top issues with AI models, as datasets can perpetuate discrimination and reinforce stereotypes based on race, gender, or other demographic and socioeconomic factors.
Lack of explainability. AI models ingest and process enormous volumes of data, and users might be unclear about how a model has parsed all that data to arrive at its findings. This closed box nature of AI models can make it difficult to identify errors – and can inhibit trust in the model’s output, even when the findings are sound.
Regulatory compliance. In March 2024, the European Union (EU) parliament approved the Artificial Intelligence Act (EU AI Act), establishing a consistent set of rules for the development and use of AI systems, with a focus on safety, transparency, and accountability. Further, the Biden administration issued an executive order in October 2023 establishing additional standards for AI safety and security, privacy protection, equity and civil rights, and responsible use of AI in healthcare and education.
Reliability and performance. As AI technology becomes more deeply embedded in business, a risk emerges of becoming dependent on systems that can break down.
A Practical Approach To Implementing AI Governance
With all the risks noted above, companies might begin to wonder – how does my organization use this technology effectively while mitigating the potential risks? The following four steps provide an outline for organizations to consider for responsible AI implementation.
Step 1: Establish an AI inventory.
- Document areas within the organization where AI technology is already in use and assign an owner to each use case. This information often is compiled via data mapping and risk assessments.
- Develop a reporting mechanism for identifying new AI use cases within the organization. This could include incorporating AI risk assessments into the third-party risk management process or developing a separate internal reporting process.
Step 2: Document AI governance policies and procedures.
- Assess the organization’s appetite and willingness to accept potential risks associated with AI deployment. Document this appetite clearly, provide examples, and communicate this risk acceptance to the rest of the organization.
- Assign a dedicated AI governance team, steering committee, or officer responsible for overseeing AI initiatives. Questions to ask include: “Who at the organization is going to take ownership of the AI technology?” and “Who do they report to?”
- Define the reporting structure for AI governance, establishing accountability and a clear escalation path for AI-related issues.
- Create a comprehensive AI policy that addresses ethical considerations, compliance with regulations, data governance, incident response plans, and transparency. Microsoft offers a publicly available resource for responsible use of AI that organizations can use as a guide in creating this type of policy.
Step 3: Implement and enforce controls.
- Document a set of controls that align with the company’s AI policy. These controls should cover areas such as data quality checks, model validation processes, and audit trails. Where possible, also implement technical controls (for example, restrict access to public AI models). The EU AI Act and National Institute of Standards and Technology AI framework can be used as guides to establish an internal control framework.
- Perform a mapping exercise to align internal controls to policies and the regulation or framework and to maintain governance coverage and control-to-regulation traceability.
- Develop a comprehensive training program for all employees who will interact with AI systems, focusing on ethical use, understanding AI outputs, and recognizing limitations.
- Provide a simple mechanism for employees to provide feedback on AI tools and processes.
Step 4: Conduct ongoing monitoring.
- Conduct periodic risk assessments of AI policies and controls in alignment with their own risk appetite and regulatory requirements, monitoring control effectiveness.
- Monitor for new and undocumented instances of AI, keeping the inventory current.
- Administer employee training on at least a yearly basis to keep employees up to date on organizational policy and regulatory changes.
- Perform regular bias and fairness testing of AI outputs.
- Assess new vendors for their use of AI technology and document use cases effectively in the organization’s AI inventory.
Embracing AI, With Caution
AI integration in the life sciences industry can open new possibilities for early disease detection, personalized treatment strategies, and accelerated drug discovery.
However, this technological revolution is not without its challenges. As AI models become more deeply embedded in our healthcare systems, it is crucial to effectively manage the associated risks of data privacy and security, bias and fairness, regulatory compliance, and reliability and performance.
By establishing a robust AI governance framework, life sciences organizations can navigate these challenges proactively, validating that AI models and systems are as secure and responsible as they are innovative.
The future of health and wellness is being reimagined, and, with careful management and strategic planning, AI tools can be a driving force in this transformation.
About The Authors:
Michael Lucas is Digital Security Principal at Crowe.
Kristen Bednarczyk is a Privacy, Data Protection and Compliance Senior Consultant at Crowe.
Luke Pillarella is a Privacy, Data Protection and Compliance Senior Consultant at Crowe.