Navigating AI's Impact On Life Sciences And Patent Law
By Noah K. Tilton
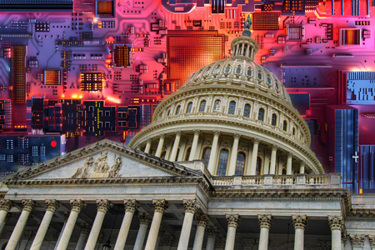
Artificial intelligence is revolutionizing the life sciences industry by driving innovation in various domains, such as drug discovery, clinical trials, and regulatory compliance. While AI offers the potential for faster and more efficient processes, it also introduces challenges for protecting intellectual property. To maintain a competitive edge, life sciences leaders must grasp how to harness the potential of AI while mitigating associated risks.
In the life sciences field, AI is fast-tracking innovation in several key areas, including:
- Gene editing: Language models are being trained to generate new CRISPR editors, enabling the editing of genomes in humans, plants, animals, and other organisms. Language models are particularly suited for sequence-based tasks due to their ability to understand protein structure and function. Other AI techniques, such as convolutional neural networks and deep learning can be employed in CRISPR-related tasks like guiding RNA design optimization and reducing off-target activity.
- Drug discovery: AI-based predictive modeling is advancing the discovery process by predicting how molecules will interact with targets. An example is the identification of Halicin, a broad-spectrum antibiotic capable of killing bacteria by disrupting their cell membranes. Researchers at MIT used in silico deep learning methods to identify Halicin, which was later validated both in vitro and in vivo. AI is also accelerating the discovery of other potential therapeutics, such as rivulariapeptolides; symplocolide A; pristinin A3; deepflavo and deepginsen.
- Medical devices: AI is playing an extensive role in designing and manufacturing medical devices and software, leading to increased efficiency, optimized supply chains, and improved product quality.
- Multi-omics analyses: AI has become a powerful tool for analyzing genome sequencing data, offering insights into patterns and identifying rare diseases. Proteomics, transcriptomics, epigenomics, and metabolomics provide valuable information at unprecedented speeds, enabling autonomous research in real-world diagnostics.
Further, foundational AI algorithms, like AlphaFold, are being developed specifically to address life sciences challenges. As AI technology proliferates across industries, the life sciences sector is actively exploring how generative AI models can revolutionize everything from drug discovery to personalized patient care.
The Intersection Of AI And Patent Law
AI implementation in life sciences raises various patent law considerations, including patent subject matter eligibility, novelty/obviousness, and sufficiency of disclosure. It is important to note that divergent examination approaches exist among different branches of the U.S. Patent & Trademark Office. For instance, patent examiners in Technology Center 1600 (“Biology and Organic Chemistry”) may advance certain rejections and claim interpretations that require different strategies than examiners in other tech centers, such as Tech Center 2100 (“Computer Architecture and Software”). Variations in application stem from the scientific and technical backgrounds of examiners, and differences in subject matter eligibility guidance, which recently underwent a further significant policy revision.
AI-based life science patent applications may face rejections under 35 U.S.C. § 101, which determines patent eligibility. Eligibility rejections in life sciences applications can be more complex than in conventional software cases, as they may involve abstract idea rejections and natural law rejections. Additionally, medical diagnostic and treatment claims present unique challenges.
Data provenance and intellectual property rights also present critical issues. Life sciences AI inventions often rely on various data sources, such as genomics data, transcriptomics data, proteomics data, metabolomics data, structural data, bioactivity data, EHR data, etc. This data is often fed into machine learning algorithms/ models (e.g., deep learning models, artificial neural networks, computer vision models, natural language processing models, etc.), which are trained or otherwise configured to generate information such as predictive biomarkers, treatment recommendations, drug efficacy, etc. Understanding and documenting issues (e.g., licensing and rights) around data sets used to train or operate AI models is essential.
Companies in the life sciences industry should also consider biometrics and user data protection laws. Given the complex landscape of AI-accelerated products, it is advisable for companies to work closely with IP counsel who possess a deep understanding of AI innovation in this space.
A Convergence Is Coming
The convergence of transformative AI and life sciences sets the stage for a new era of innovation. Early adoption is shown to disproportionately benefit individual researchers and companies by outperforming their peers. As AI matures, and the focus shifts toward more sophisticated AI pipelines, such as “test-time scaling” — which provides additional computation during the inference phase — this area is anticipated to experience significant growth.
About The Author:
Noah K. Tilton is a partner and patent attorney with the Chicago-based intellectual property boutique law firm Marshall Gerstein. Noah has significant experience working closely with those innovating at the intersection of computation and life sciences.
DISCLAIMER: The information contained in this article is for informational purposes only and is not legal advice or a substitute for obtaining legal advice from an attorney. Views expressed are those of the author and are not to be attributed to Marshall, Gerstein & Borun LLP or any of its former, present, or future clients.