'Explainambiguity:' When What You Think Is Not What You Get
By Vincenzo Gioia and Remco Jan Geukes Foppen
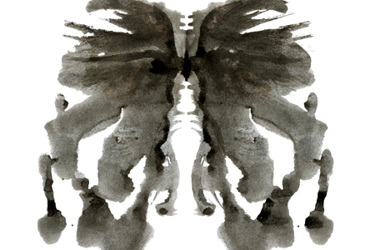
The rapid adoption of AI in the pharmaceutical market has led to a revolution in the research process and pharmaceutical development techniques. The AI revolution is not free of risks, however. This is why the EU AI Act and President Biden's 2023 Executive Order on AI took effect highlighting the global importance of secure and trustworthy AI development and use.
Regulatory agencies like the FDA and EMA have anticipated these frameworks by issuing guidelines for AI use in clinical trials. The FDA's CDER has seen a notable increase in IND submissions with AI components, indicating market responsiveness. While regulatory agencies focus mainly on transparency, such as data for model training or specific modeling techniques, there's growing attention on explainability in AI (xAI). xAI is becoming a crucial element for the implementation of AI systems for drug testing and development. However, the concept of "explainability" presents significant ambiguities, both from a linguistic and technological point of view.
What Is Explainable AI?
Explainable AI (xAI) refers to a set of statistical methods and processes that allow users to understand and describe AI models. This seems straightforward. However, there is ambiguity about how to define explainability, its uses, its differences, and its relationships with guidelines. Despite this, we can say that xAI enables the review of AI-generated results, focusing on both outcome and model explanations. Explainability methods can be categorized into three main dimensions:
- Specificity: Model-agnostic vs. Model-specific
- Application Time: Intrinsic vs. Post-hoc
- Scope: Global vs. Local
Model-agnostic and post-hoc xAI methods can be easily applied to both local and global approaches. Post-hoc methods generally can be implemented with minimal modifications. In contrast, model-specific or intrinsic methods often require more complex adaptations.
What Does Explainable AI Deliver?
xAI is designed to help decision makers and regulators build trust in AI outcomes, with a primary focus on explaining those outcomes. This offers substantial business advantage because global explanations typically support strategic decisions (such as training vs. staffing, building, or buying). Meanwhile, personalization and segmentation efforts, such as patient stratification and adverse outcome prediction, benefit from local explanations. Some xAI models reveal the training data that influenced specific parameters, while others quantify the impact or provide the directionality of that influence.
Explainability In The Boardroom
The operational impact of xAI is substantial, especially as 37% of the market views explaining results from GenAI algorithms as a strategic priority that goes beyond regulatory compliance. xAI presents a strategic opportunity to enhance transparency in decision-making, build stronger stakeholder trust, and detect potential — even tolerable — biases in AI models. Furthermore, implementing xAI can improve risk management by enabling quicker identification and correction of model errors, which is particularly vital in the pharmaceutical sector, where AI-driven decisions directly impact public health.
Guidance and frameworks for specific uses of Al and drug development (EMA reflection paper and FDA discussion paper) and xAI are connected, even if the emphasis is on transparency. For now, in the interconnected system of laws, regulations, and guidances, some geographies are applying hard laws and others are taking a risk-based approach toward AI oversight.
Concerning the global drug development endeavor, applying explainability globally and cross-functionally could be competitively disadvantageous in local markets unless xAI proves to be a source of differentiation, where xAI capabilities will win the trust of regulators and enhance ‘what if’ capabilities. In that case, xAI can help developers and regulators to anticipate and prepare for contingent situations with more precision. Alternatively, applying explainability per use case would require multiple explainability standards that will likely be more complex and costly.
Ambiguous Nature Of Explainability
Explainability is meant to address ambiguity. However, explainability has some ambiguities of its own. When we talk about xAI we have two different problems. The first is related to the linguistic meaning of the word and the second depends on our way of thinking about a tool.
The “linguistic-explainambiguity” that arises when talking about xAI is related to the common use of the term "explainable" and its meaning in the context of AI. In everyday language, an explanation generally involves the identification of cause-effect relationships that govern a phenomenon. In the context of AI, however, the term refers to a probabilistic representation of the internal dynamics of a model, without necessarily entering into a causal analysis.
The “technology-explainambiguity” is related to the way we think about a tool that is usually identified as a single object developed to do a specific thing. When we talk about xAI, it refers to a set of statistical methods and processes designed to capture only one aspect of the overall concept.
Different Explainabilities For Different Contexts
This diversity in application complexity demonstrates that "explainability" encompasses a range of approaches and techniques, and takes us to a question: “If explainability is not a universal term, do various contexts require different levels of explanations?” To better understand the practical implications of this question, let's examine two use cases specifically used in the pharmaceutical industry: Search in large libraries of data, and disease prediction and patient stratification.
Search in large libraries of data is a model designed to search and extract data from documents and generate a document based on the found data. This model provides a detailed list of sources used for each section of the paper, thus offering a form of traceability and explainability. For disease prediction, explainability focuses on understanding the factors that influence the prediction and their relative weight in the model's decision-making process.
Use Case: Search In Large Libraries Of Data
Scanning and searching biomedical literature along the broader drug development pipeline is an important and time-consuming activity. Large language models can be trained to predict therapeutic targets or disease prevalence in patient populations for better patient stratification in clinical trials. An AI-based automation of this activity offers significant benefits, such as cost reduction, time efficiency, and minimizing the risk of missing relevant literature. But how can we be sure that the collected data is free of hallucination? The solution is part of the AI model that is developed with an intrinsic xAI module that lists the exact source document or even the specific sentence where data was found. This ensures the authenticity of the information and allows the user to give feedback about the quality of the correct data source identification. Following this, reinforcement learning based on human feedback can add a degree of confidence to the literature search, directing future searches better. This technique is a trustworthy solution to the problem of scanning biomedical literature where references to documents are pointed out. This is short of explaining the decision itself.
Use Case: Disease Predictions And Patient Stratification
Predicting heart disease, a leading cause of mortality worldwide is crucial for early diagnosis and treatment. AI empowers the analysis of vast clinical datasets, uncovering patterns and features that can be difficult to detect through traditional methods. A relatively new trend in disease prediction is the application of explainability that ranks the features by importance (Fig 1 left). Furthermore, individualized patient-specific risk factors can be identified from differences in outcomes in order to improve patient stratification in clinical trials (Fig 1 right).
Fig 1. SHAP (SHapley Additive exPlanations) analysis of heart disease dataset: An anonymised dataset was collected to predict whether an individual was suffering from heart disease. The labeled dataset consists of 14 attributes (sex, chest pain type, resting blood pressure, serum cholesterol, fasting blood sugar, resting ECG results, max heart rate, exercise induced angina, ST depression due to exercise, age, slope of exercise ST segment, # of major vessels, Thalassemia disorder presence, the predicted attribute) from > 300 patients, and was analyzed using a supervised machine learning model (XGBoost). The AI model prioritizes the importance of every attribute to define the prediction, using SHAP. The global feature importance plot, i.e. the disease-level explanation (Fig 1 left), shows that cp (chest pain) is the most important feature, followed by thall (Thalassemia disorder), and caa (number of major vessels colored by fluoroscopy) were identified as significant predictors in the Cleveland dataset, aligning with clinical understandings of their roles in heart disease. The right plot is a local feature importance plot (i.e. patient-level explanation), where the bars are the SHAP values for each feature, that shows the features’ importance ranking and its directionality for a single patient. Clustering tasks could be adopted to identify sub-groups of patients.
However, even with a reliable dataset, how can we ensure the quality of the reasoning behind an AI model's decisions? The solution lies beyond the AI itself, in a set of tools derived from mathematics and economics. One such tool is the SHAP library, which provides a detailed breakdown of the significance of each attribute and their interdependencies. While this technique offers a level of trustworthiness, it is not foolproof. A SHAP explanation offers a probabilistic interpretation of the statistical patterns that drive the AI model's output.
Explainambiguity In A Risk-Based Framework
The high-risk strategic decisions (risk to patient and regulatory decisions) and the intended audience would indicate the level of explainability. Regulatory authorities would probably require a higher level of explainability in this risk-based framework. The two use cases put levels and meanings of explainability on display. The first use case gives a partial explanation of the AI model output, which is a good representation of the linguistic-explainambiguity of the xAI tools. The second use case is a technology-explainambiguity that stems from the various interpretations of the term "explanation," which can differ in context. In low risk cases, of course, explainability would not be adopted, and then ambiguity would not be a factor. The requirement for levels also depends on real-world use and computing power. Structured prompt engineering will strengthen a LLM response, but real-world use will probably cause suboptimal prompt engineering. Therefore a higher level of xAI could provide an additional safety net. Alternatively, a reason to reduce the level of explainability could be when using a biomedical data set of billions of features where the computing power is too limited to address this high complexity.
Explainability Is Moving Towards Disambiguity
In conclusion, as AI continues to transform the pharmaceutical industry, xAI emerges as a key element to ensure the reliability, transparency, and effectiveness of these advanced systems. Business leaders must be aware of the challenges and opportunities presented by xAI, promoting a balanced approach that facilitates the potential of AI while maintaining the safety and ethical standards required in the pharmaceutical industry.
About The Authors:
Remco Jan Geukes Foppen Ph.D., is an AI and life sciences expert. He is sensitive to the impact of AI on business strategy and decision-making processes. Remco is an international business executive with proven expertise in the life science and pharma industry. He led commercial and business initiatives in image analysis, data management, bioinformatics, clinical trial data analysis using machine learning, and federated learning for a variety of companies. He has a Ph.D. in biology and holds a master’s degree in chemistry, both at the University of Amsterdam.
Vincenzo Gioia is an AI innovation strategist. He is a business and technology executive, with a 20-year focus on quality and precision for the commercialization of innovative tools. Vincenzo specializes in artificial intelligence applied to image analysis, business intelligence, and excellence. His focus on the human element of technology applications has led to high rates of solution implementation. He holds a master’s degree from the University of Salerno in political sciences and marketing.