Google Life Sciences Leader Talks AI Opportunities And 'Watch Outs' For Biopharma

By Ben Comer, Chief Editor, Life Science Leader
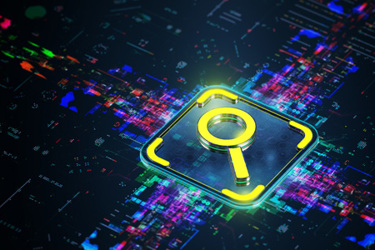
Google’s global market leader for life sciences, Shweta Maniar, spoke with Life Science Leader about how large and small biopharmaceutical companies are using AI to lower costs and expedite timelines. Maniar, who currently sits on several industry boards and has previously worked as a scientific researcher, and as a commercial partnering and strategy principal at Genentech, described Google’s biopharma clients as “strategic partners,” and emphasized the importance of mutual collaboration. “It's about meeting the pharmaceutical and biotech industries where they are, so that we're actually supporting their endeavors,” says Maniar. This Q&A has been condensed and edited for length and clarity.
Can you give me a sense, and maybe a couple of representative examples, of how biopharma companies are using Google Cloud and AI tools right now?
A lot of effort is also being put into improving the design of clinical trials, but not just the design, it’s also recruitment, and the analysis on the other end. And it's not just about interacting with a pharma company, it's also how do we connect in with the CROs and become a part of the full cycle. Looking ahead, generative AI models are going to be able to design brand new molecules with specific therapeutic properties. That’s futuristic, but it does have the potential to unlock entirely new drug classes and treatment options. We're seeing that generative AI has this potential to really reduce the time and cost of bringing therapies to market.
Regarding use cases, I'll break it up into a couple of biotech and pharma-specific examples. Biotech has really been looking at drug interaction predictions; how you can use these different models to predict how different drugs are interacting with each other within the human body. This helps scientists and researchers design safer and more effective drug combinations. Along with that, another use case is around molecule generation. Many organizations are focused on how you can design novel molecules with the exact, desired properties, such as high potency and sensitivity for the exact targets that you're looking for. This is the idea of being able to look at completely new classes of drugs.
From a pharmaceutical perspective, there’s drug repurposing. I have this portfolio, how do I do more with what I have? Organizations are using generative AI to analyze existing drugs and identify potential new uses based on their mechanism of action. Another example I'm particularly very keen about is the idea of overcoming data limitations in the pharmaceutical space using synthetic data. Generative AI can generate synthetic data to augment some of the limited real world data sets, to improve the accuracy and the robustness of these AI models. As more organizations move into the rare disease space, synthetic data can be used to predict a patient response to treatment. In the clinical trial context, synthetic data may be useful in identifying trial participants, and then using generative AI to streamline some of the clinical trial processes. Theoretically, success rates can be improved because you are identifying the appropriate cohorts to begin with. I'm talking about this in the pharmaceutical context, but the use of synthetic data is also pretty applicable on the device side as well.
Is there an example you can give on how synthetic data might be used in the context of rare disease R&D?
SM: I’m not able to speak specifically around the drug discovery piece, because this is a lot of the work that we're doing with some organizations that are in process right now. But I can give you a synthetic data example in what we’re doing with Bayer. Bayer is leveraging AI to supercharge its drug discovery efforts. They are analyzing massive data sets, and they're automating a lot of their tasks. When they initially started to work with us, it was Bayer working with Google Cloud with the idea of being able to support their developers from the initial idea of deployment to AI-powered healthcare solutions and applications. That was pretty broad to begin with, but now what we have done is we've actually identified use cases where we're working with some of the synthetic data. Bayer is already creating synthetic images for oncology based on histology images. That's really a big deal, because it's overcoming that same limited data that's available for these for training algorithms, especially when it comes to their rare disease portfolio. Bayer’s use of synthetic images has actually proven really valuable, both in the oncology setting as well as in the radiology parts of their business. It's a very interesting use case for synthetic data, and particularly in imaging in this case.
Last year, Google announced the launch of a target and lead identification suite, which Pfizer and Cerevel Therapeutics are using, and separately, a multiomics suite, which Colossal Biosciences is using. Thinking about small and midsized biotech, how do companies begin the journey into AI adoption?
SM: A lot of what you saw announced last year is around taking our capabilities and creating these offerings in collaboration with our partners, be that the target and lead ID with the use of AlphaFold 2, or even the multiomic solution suite — it was all to support our partners’ endeavors. Superluminal Medicines is a smaller sized organization that is also working with Google Cloud. Superluminal Medicines is well known for accelerating its drug discovery processes, where they're focused particularly on creating candidate-ready compounds that target G protein-coupled receptors, or GPCRs, with small molecule therapeutics. Their unique approach to modeling proteins and drug interactions sets them apart. Proteins are always in motion and Superluminal understands that capturing the dynamic range is crucial for an effective study. They are harnessing Google Cloud's compute power, and they’re analyzing multiple protein structures and combining them to generate a dynamic protein model. It’s like combining individual photos to create a movie; it looks like a continuous, moving image. Superluminal is using that same thought process and creating these dynamic models that give researchers much more of an accurate picture of how these proteins function within the cell in the body. It’s a prime example of the work that they're doing to expand on AlphaFold 2. They've taken our solutions and capabilities and have even gone beyond sequence to structure, to generate multiple confirmations or shapes. The idea is they now can enhance the publicly available foundation models based on their expertise, and the applications that they need from Google.
On target and lead ID, Chugai Pharmaceutical Co., a midsized pharmaceutical organization based in Japan, and part of Roche but functioning relatively autonomously, is looking at using Deepmind's AlphaFold 2 and the target and lead ID for its protein prediction tool. Because we've created this offering for the ecosystem, it's not just around leveraging a research tool, but by using Google Cloud's Vertex AI, it becomes much more of a turnkey, scalable, and flexible solution. That makes it much easier for organizations like Chugai to implement these solutions into their existing systems.
We're at a pivotal moment in our AI journey. Breakthroughs in generative AI are fundamentally changing how people are interacting with the technology, and frankly, we're learning from our partners and our customers about additional use cases as we're going through these journeys together. It really is just the beginning, but we've prepped for this moment.
The FDA and EMA have both published guidance around AI frameworks and the need for certain amounts of explainability. Does Google incorporate regulatory guidance into the development of new AI tools?
SM: We, as Google, are often called upon by regulatory bodies to provide our perspective on how we are looking at the development of AI, to inform the FDA and other regulatory bodies’ perspectives on how they're going to be putting out their guidance. To be very clear, that is not to say that we are telling them what to do. We're providing Google's perspective, and I appreciate that they are looking openly and trying to understand the implications of AI, how they should be thinking about it, and what to understand in the near-term and in the future. We are continuing to have dialogue with these regulatory bodies.
Should biopharmaceutical companies experimenting with AI begin with explainability in terms of building a model or building a use case, or should they first identify a use case and then work backward to determine and present explainability?
SM: Prioritizing explainability is going to be paramount. I don't know if there's a right order in the way that you've set it out, but it really is important to identify your problem clearly, and to choose the right AI framework so that it has transparency and interoperability, and being able to understand how you arrived at a given output. Education is important here, because you don't have to be an AI expert to also be expected to understand the outputs of these models. This is not a black box exercise. We should be able to have a full explanation for what these organizations are trying to do. We cannot expect to just follow a model just as it is. We do need to have some level of transparency as different pharmaceutical organizations are going through the process. For any project that a pharma company engages with, it is part of that responsibility to help think through the explainability.
What are the biggest or most frequent mistakes that biopharma companies make in trying to integrate new AI tools or solutions into their organizations?
SM: This is not unique to generative AI specifically, but to any AI project: organizations must have a very clear strategy. Someone might say, proverbially, ‘I'm looking for a hammer and a nail.’ No, you're looking for a hole in the wall. What is it that you're trying to achieve? It shouldn't be buried in your 153-page deck. You should be able to articulate it in a couple of sentences. That's the first 'watch out:' be very clear about what your strategy is and what you're trying to achieve. Another challenge is an organization might have a key problem, but maybe it’s not a data problem. Organizations have to ensure that they have the right amount of data, or make sure that they don't have insufficient data, or insufficient data quality and management. I would also come back to your comment around explainability. Organizations cannot ignore explainability and transparency; there always has to be a level of accountability and trustworthiness. As organizations are going through their own processes internally, I think that's a big 'watch out:' making sure that everyone can fully understand, in a nontechnical way, what you're going after.
There is also an important cultural aspect. People might think, for example, that they are not the technical expert, the AI expert, so they don’t need to fully understand the process. In reality, we all have a responsibility as the industry moves forward, no matter what our role is in the organization. There is a lot of excitement about what AI can do, and I think it becomes incumbent upon everyone in the industry to try and educate themselves and make sure that they understand it. This brings me back to my earlier comment that we are just at the beginning. We're on a journey together, and this is why I very intentionally say biotech and pharmaceutical organizations are not just customers. They are strategic partners, because we are going through the process together and learning. There is a lot of back and forth, because it's not just about the technology. It's about meeting the pharmaceutical and biotech industries where they are, so that we're actually supporting their endeavors. It's not one size fits all.